Three considerations for the future of AI
By GovInsider
Experts from IHiS and SG Enable discussed at Singapore Computer Society’s ‘Impacting Society With AI’ panel.
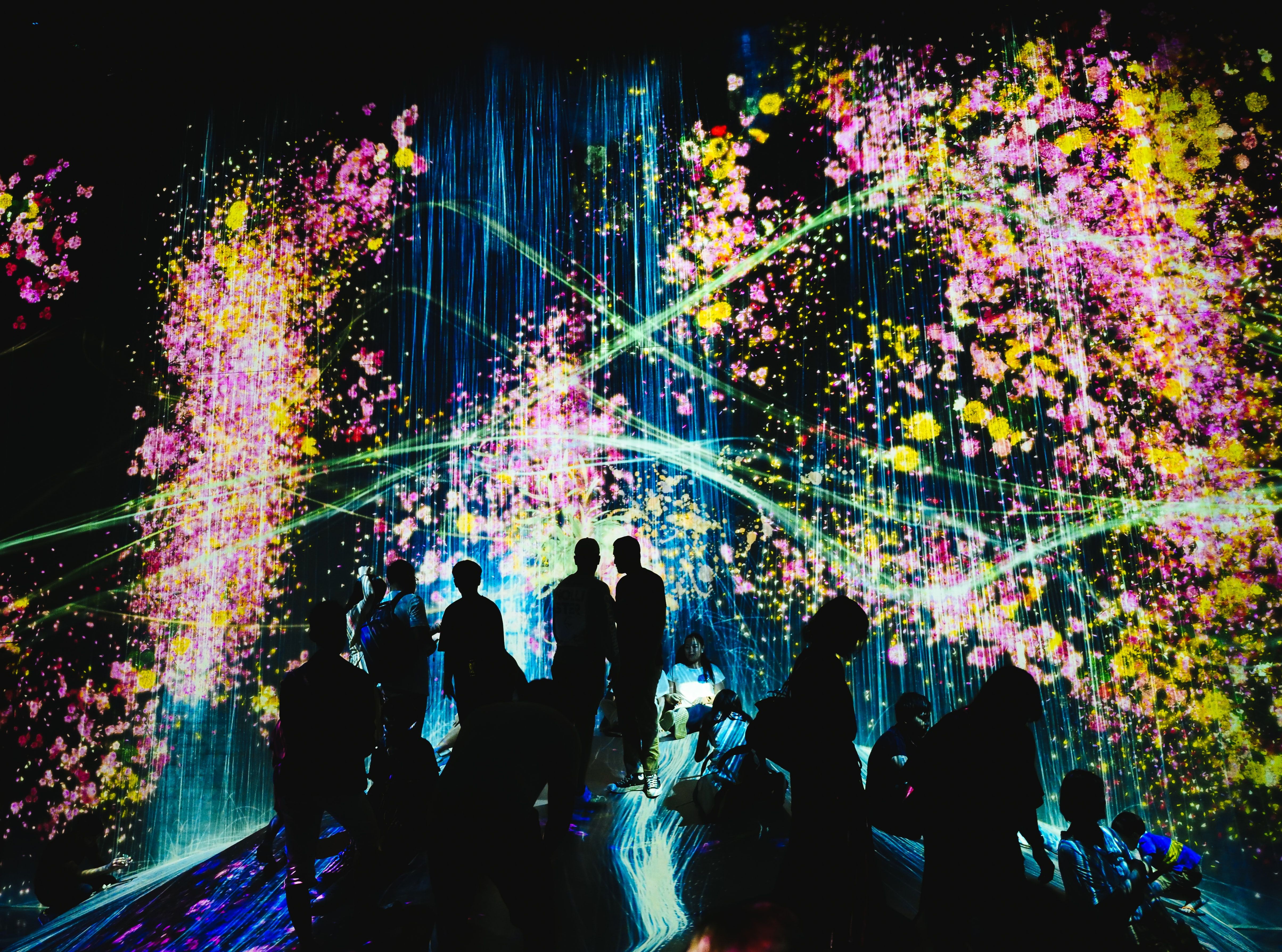
“I am to convince as many human beings as possible not to be afraid of me,” wrote a robot for The Guardian’s op-ed. The robot, GPT-3, used machine learning to write the text - and editing it took less time compared to many human op-eds, The Guardian revealed.
Many have contemplated the perils of artificial intelligence. Stephen Hawking warned it would “spell the end of the human race”. But the technology has also brought benefits aplenty, especially during Covid-19. Singapore has used it to build a temperature scanning tool in two weeks, and predict manpower bottlenecks in its infectious disease response centre.
How can healthcare organisations reap the benefits of AI while guarding against its downfalls? Experts from IHiS and SG Enable discussed at the Singapore Computer Society’s ‘Impacting Society With AI’ panel.
1. Eliminating bias
AI learns to make decisions based on data, which can include biased human decisions or social inequalities. This is a crucial problem as organisations plan to deploy AI rapidly, scaling such biases. Amazon scrapped its AI recruiting tool in 2018 after they discovered a bias against women, Reuters reported.
The model was trained to vet resumes submitted over a 10-year period, most of which were from men. So it taught itself to penalise resumes with the word “women’s” - and favoured those with commonly used language by males, such as “executed” or “captured”.
AI might also “unknowingly” leave out people with disabilities, said Justin Seow, a tech analyst (Enablers Development) at SG Enable. Some organisations use filtering systems based on past successful hires to scan through resumes. This could exclude people with disabilities who might not have had employment opportunities previously, he added.
It’s essential to recognise AI’s limitations, said Christine Ang, Deputy Director of IHiS’ Emerging Capabilities-Health Insights. A “clear set of measured outcomes” and constant reviews must be in place to reveal any hints of bias, she added. IHiS tested its AI temperature scanning tool on 3,000 faces before it was made available as a pilot. Its CEO said: “The more we trial it, we’re bound to find some deviations, it will help us to refine the AI model.”
2. The interpretability problem
With the help of deep learning, AI is now able to predict new ways to synthesise molecules and even predict future crimes. But developers don’t understand how some of the algorithms work. Data is crammed in on one end - and results come out of the other - but no one really knows what happens in the middle.
In 2015, the Mount Sinai Hospital in New York trained an AI model using data from about 700,000 patients. Without human instruction, the algorithm was able to discover hidden patterns that indicated if someone was at risk of schizophrenia. The psychiatric disorder is notoriously hard to detect, and physicians still don’t understand how the model predicts it.
This poses a huge problem as doctors have to provide patients with a rationale behind the diagnosis. Explainable AI still remains the”most challenging area in terms of machine learning”, said Ang. “In healthcare, we can mitigate some of this by doing cross checks against established manual norms,” she adds.
IHiS tests its AI models with a local population data set before it is executed.In the long run, the healthtech agency aims to build clinicians’ ability to explain how certain medical conclusions were derived, she added.
3. Privacy and security
As the use of AI accelerates, it magnifies the ability to use personal information in ways that will violate data privacy. Data privacy is of “utmost importance” in healthcare, and IHiS has to think of new ways to protect the patient's confidentiality, said Ang. Singapore’s National University Health system has built a data crunching platform which anonymises data for the testing of AI models.
It all “boils down to consent”, said Seow. SGEnable uses an AI visual attachment device to help the visually impaired identify text and recognise faces. After obtaining consent, the device provides its users with the name and gender of who’s in front of them, he added. To Ang, transparency is key.
The use of such technology should be “controlled to a specific use case” and made known to the public, she said. “You need to know what you're signing up for.” Artificial intelligence wields an immense potential to enhance patient care and help organisations create inclusive services. Before that can happen, however, organisations must resolve issues around privacy and bias.