Sustaining AI boom in Singapore’s public healthcare
Oleh Red Hat
The country’s public healthcare sector is booming with AI innovations and developments, but what enablers will be necessary to sustain this energy? A closed-door roundtable at GovInsider Live Healthcare Day tackled this question.
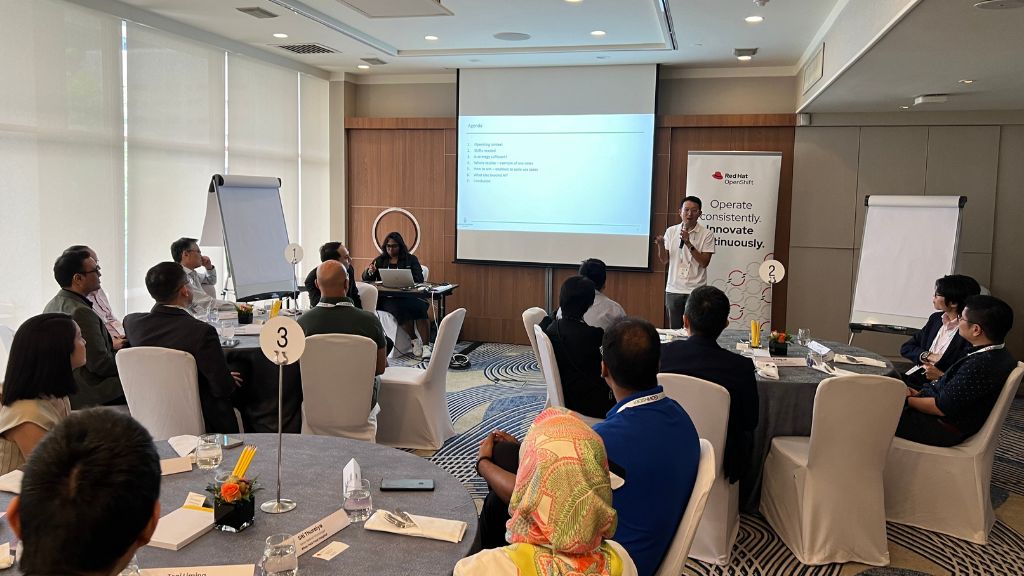
At GovInsider Live Healthcare Day, participants at a closed-door roundtable discussed strategies to sustain AI innovation in Singapore's public healthcare. Image: GovInsider
From an app that transcribes and summarises conversations with patients in real time to a tool that can generate “visual pillboxes” to remind senior citizens of their pill regimens, Singapore’s public health system is seeing a boom in artificial intelligence (AI) innovation.
Such AI tools promise to help healthcare staff raise productivity, better engage patients, and produce new health solutions as the country tackles challenges such as an ageing population, rising medical costs, and a healthcare labour crunch.
But if not managed carefully, booms can often turn into busts. As the hype cycle dies down, innovators become bogged down in tedious funding and regulatory cycles, and attention turns to business-as-usual tasks.
At GovInsider Live Healthcare Day, healthcare professionals discussed how policymakers and tech leaders can streamline AI innovation and sustain current energy to solve the wicked problems of tomorrow at a roundtable.
To subscribe to the GovInsider bulletin click here.
Scaling innovation with a clear strategy
The session kickstarted with a presentation by the Ministry of Health’s Director of Health Analytics Division, Sutowo Wong, who spoke about the key enablers to scale AI innovation in the years to come and the importance of developing a clear strategy.
“Strategy is about choices: Where do we play? How do we win? Essentially, where do you want to place your bets, what are the use cases, and are you aligned with the overall objective of the organisation?” he said.
He highlighted that even as the health ecosystem works on pilots, it is important to identify which ones to prioritise, how to minimise duplication, and ensure that such pilots can be integrated with existing IT systems.
Once prototypes have been developed, there should be enablers in place to support scaling, such as funding, digital infrastructure, and data availability. For instance, the MOH Health Innovation Fund is one such effort that provides financial support for projects aligned with broader health system goals, he noted.
Other enablers include raising data competencies across the healthcare sector and making sure that projects have access to critical talent, such as data scientists, AI engineers, UX designers, and business folks, that can provide well-rounded perspectives.
It is also important to translate the principles set out in AI in Healthcare Guidelines into specific actions that projects should take to ensure safe and responsible use of AI.
He also highlighted the importance of creating a data-driven culture within organisations and securing the buy-in of senior management to support AI innovation.
“Most importantly, it’s not just within our organisation, it’s about working together. Having technologies e.g. Privacy Enhancing Technologies (PET) that allow us to collaborate securely with one another is also important.”
A platform approach to support collaboration
Red Hat’s Asia-Pacific Head of AI, Sandip Sharma, shared how a common scalable platform can enable collaboration across the various stakeholders in a healthcare ecosystem.
He began by discussing the many ways in which AI is helping doctors with decision-making and highlighted the case of Boston Children’s Hospital, which has developed an AI application that can automatically examine X-Rays, identify the most valuable images, and flag warning signs to radiologists.
As it is built on Red Hat OpenShift, the hospital can immediately share the application to public research clouds to support collaboration and further innovation.
“They have built a fantastic algorithm, but the problem is how they can share this. That’s exactly what they are using Red Hat OpenShift for, to share this extremely useful algorithm across the world in a very secure and scalable fashion,” he said.
It is critical for tech leaders to build a hybrid cloud platform that can enable consistent data sharing across complex cloud environments, ensure data interoperability, and ensure the platform is compliant with regulatory standards to enable innovation, he explained.
Singapore’s Synapxe, the country’s national healthtech agency, has recently unveiled TANDEM, a secure platform for developing generative AI (GenAI) applications, and HEALIX, a cloud-based analytics platform that connects public health data sources and provides tools for building AI models.
To subscribe to the GovInsider bulletin click here.
Confronting data challenges
During the roundtable discussions, healthcare professionals and policymakers discussed some of the key challenges hindering AI innovation today and potential steps forward.
Many of the challenges raised had to do with concerns over data, such as ensuring that such innovations are compliant to data protection and compliance regulations, implementing responsible AI governance and ethics principles, data residency requirements, and accessing data held by other stakeholders.
Participants also highlighted that other stakeholders such as private healthcare players and community partners may have limited resources to take part in such projects and collaborate meaningfully.
Sustaining AI innovation also requires agencies to move away from traditional waterfall methods of development – instead, healthtech will have to embrace a “build fast, fail fast” mentality, but tech leaders will have to convince management that such agile methodologies will not be harmful.
To tackle these challenges, the participants said it was important to closely collaborate with regulators to ensure compliance, establish robust data security measures from the pilot stage, and encourage best practices for AI development, such as a human-in-the-loop approach and monitoring model performance.