Using behavioural analytics to look for cyber threats
By Micro Focus
Cybersecurity experts from Micro Focus explain how AI & ML can study entity behaviour to find masquerading threat actors.
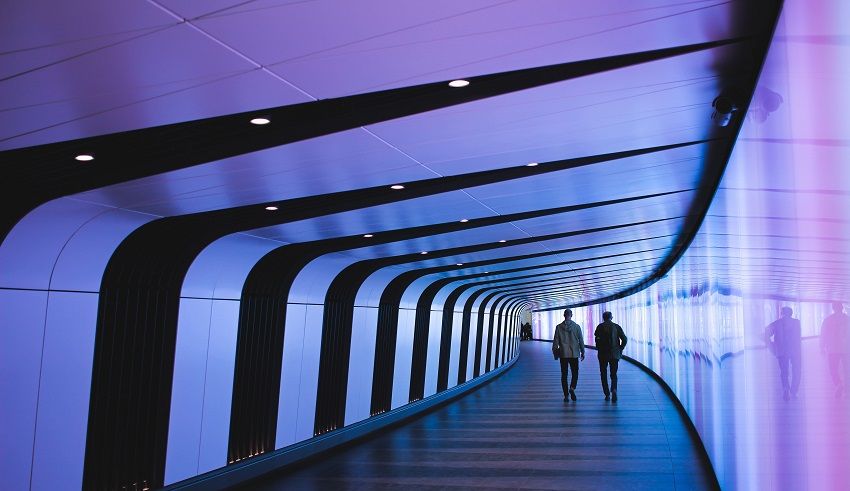
With so much noise online, how can organisations understand what’s going on in their networks, find the real threats and shut them down? Cybersecurity experts at Micro Focus are turning to behavioural analytics with the use of ML and AI.
They explain how studying each entity behaviour and creating a baseline can help to reduce false alarms, and why this is more effective than the traditional binary approach to risk detection.
Risks with Covid-19 and remote working
The pandemic and remote working have introduced two main risks online. First, employees may be more vulnerable to cyber attacks now because of the fear and uncertainty surrounding the pandemic. Scammers have sent out phishing emails impersonating the World Health Organisation, for instance, promising relief funds or asking for donations.
Second, remote working means more authentications. Companies employing appropriate safeguards may get employees to verify their identities every time they access the company network.
This generates a lot of data, however, which might bury any suspicious activity. Typically, security teams have to go through each log, select suspicious ones to investigate and assess which ones to shut down. With so much data, it could take teams days to zoom in on anomalies, and even then, it is only a hit and miss approach.
Why the current AI/ML approach doesn’t work
Many cybersecurity tools on the market are already using AI to sieve out anomalous activity. Most of these are rules-based, which means that they are programmed to respond a certain way every time it encounters the same trigger. This approach is useful for detecting malware - each time the algorithm finds a specific pattern, it knows to block the malicious code from the network.
But this doesn’t always work. A rigid, rules-based approach only tells us that something is potentially a threat because a rule has been broken, not if it is unusual enough to investigate.
For instance, security teams might programme a rules-based AI model to raise an alert when employees log into the system outside of office hours. With remote working and flexible hours, some employees might choose to work late into the night. The AI model doesn’t take into account context to understand why an employee’s behaviour might have changed, and could potentially raise a lot of false alarms.
A better approach
One way around this is to understand each employee’s typical behaviour, and to constantly compare anomalous activity to this baseline. Micro Focus’ ArcSight Intelligence solution uses non-rules-based unsupervised machine learning to study employee (entity) behaviour and create an individual baseline for every entity.
The algorithm doesn’t ascribe a binary ‘good’ or ‘bad’ label to an employee’s behaviour. Rather than highlighting every non-ideal behaviour as dangerous, it can learn why someone’s behaviour might be unusual, and then decide whether it really is malicious or not.
This is also useful for flagging when an employee does something they’re authorised to, but is unusual for them, such as downloading a lot of data or attaching large files in an email. These would not be flagged by a rules-based algorithm, but they can be a good sign of insider threats, or of external threats masking as employees.
Security teams might sometimes create behavioural baselines for an entire department, instead of individually. Two people from the same department may have different access behaviours, so this approach is not the most helpful.
ArcSight Intelligence's mathematically based algorithms look at all types of behaviour to find the individual baseline, including how an employee usually interacts with shared folders on Monday mornings, or how much data they attach in an email. The more data it collects, the more accurate the baseline becomes. These baselines can also be broken down into more specific time periods to show how an employee behaves within a quarter, or within a day.
It’s important to take all of a person’s interactions and behaviours into account, rather than looking at each suspicious activity in isolation. If an employee has done something suspicious before, their subsequent suspicious behaviours will be considered much more risky. This will be reflected in the risk score that ArcSight Intelligence calculates for every staff.
AI, with the use of unsupervised ML, is an exciting weapon for cyber threats, but there is a lot more it can do to identify real threats. With the spike in criminal cyber activity, behavioural analytics will help security teams get an accurate picture of everything going on in their networks.
Request for a free live demo of Micro Focus’ ArcSight Intelligence here. For other product inquiries, connect with Micro Focus here.