Three governments using data to fight epidemics
By GovInsider
How are Singapore, Indonesia and America tackling malaria, dengue and West Nile Virus?
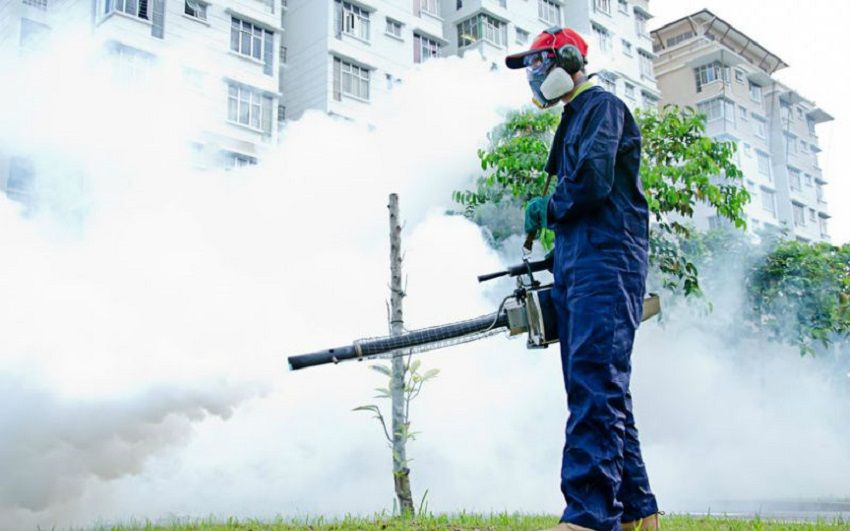
The mosquito is more than an annoying pest - it represents a serious public health threat all over the world. To prevent mosquito-borne disease outbreaks, governments are turning to data and machine learning.
Complex analytics models can predict where mosquitos will strike, and allow preventative campaigns against them. GovInsider has looked at how Singapore, Indonesia and the US are implementing risk maps, predictive models and reporting systems in their strategies to defeat this elusive adversary.
1. The United States
The city of Chicago, Illinois in the US has built a predictive model that shows a week in advance whether there will be West Nile Virus (WNV)-carrying mosquitoes in a particular area.
The Chicago Department for Public Health (CDPH) teamed up with the Department of Innovation and Technology (DOIT) to build this model, which allows them to carry out targeted mosquito-spraying in the areas most at risk.
Despite the fact that the most abundant mosquito species in Chicago are not carriers of the disease, WNV remains a threat. This is especially so as the climate warms and mosquito populations increase.
In 2002, there were 225 human cases of WNV in Chicago, which included 22 fatalities - the worst epidemic yet for the city. In 2015, the city government launched a competition for data scientists to develop predictive models for the WNV problem.
To aid the competitors, the government published data such as mosquito spraying programme locations, schedules, historical records of WNV detection at trap sites, and local weather data on its open data portal.
The model now being used by the city government predicts whether a CDPH mosquito trap will have WNV present on a given trap collection week.
This is important, because current control measures include mosquito spraying in areas when mosquito traps test positive for WNV two weeks in a row.
“Given this week’s results, can we predict if there will be WNV at a given trap location next week? Building an algorithm that can detect at-risk sites earlier means that we can spray sooner, reducing public exposure to the virus,” Gene Leynes, a senior Data Scientist at DOIT, was quoted as saying.
2. Singapore
Dengue is a seasonal threat in Singapore, peaking in the warmer months of June to October. The government carries out preventive surveillance of dengue hotspots to control this threat, but this requires skilled officers to physically check these locations, draining already limited resources.
The National Environment Agency came up with a risk map that draws from data to identify the areas most at risk of dengue, both on a town- and city-wide level. This map allows the agency to deploy its limited number of officers more effectively.
The machine learning model draws from data such as past dengue cases, vegetation indices, public transport information, and mosquito population data to create a colour-coded map. The agency now uses this map to plan resource allocation.
3. Indonesia
In Indonesia, the South Halmahera Malaria Centre (SHMC) collaborated with Pulse Lab Jakarta (PLJ) to develop a mobile phone-based malaria reporting system which effectively sped up malaria response by 19 days.
To give you some context, South Halmahera is made up of a cluster of islands with unreliable electricity and spotty Internet. This means that online solutions are out of the question.
Furthermore, reports on malaria cases are compiled by hand, require a costly journey by boat to deliver, and get to the SHMC once a month at best. With the mobile phone-based system, users simply text dedicated phone numbers to track patients or medical stocks.
A simple dashboard then aggregates and visualises this data. The system effectively reduced the time taken to report on cases, making it much more responsive for the treatment of malaria.
In fact, this is the first time that a mobile phone-based system has been implemented to improve malaria reporting in Indonesia. Fighting these diseases doesn’t have to use the most cutting-edge technologies.
It just requires an effort to gather accurate data, and to use this in the decision-making process. As doctors are finding across the world, every data set counts.
Main image from Gov.sg; map images from DOIT and NEA