AI holds both promise and worry for cybersecurity
By Amit Roy Choudhury
AI allows for tracking all elements within rapidly expanding networks; it also allows hackers to use increasingly sophisticated attack vectors.
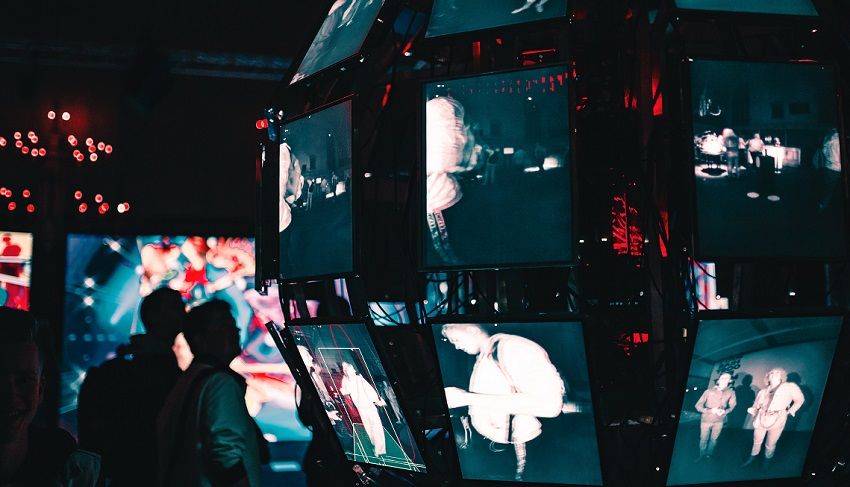
The network boundary has become fuzzy and continues to grow and evolve rapidly. Typically, a large enterprise with suppliers, customers, and other stakeholders logging on to their network for ease of doing business, needs to have the ability to analyse several hundred billion time-varying signals accurately every day to calculate the risk of a breach. For public sector organisations that provide citizen-centric services online, like tax portals or provident fund boards, the number of hits or signals that try to communicate with the server could be even higher.
While automation of security tasks has been a feature for some time, it is no longer possible to keep track of such high-volume transactions or hits with traditional cybersecurity tools. Information security professionals are increasingly turning to Artificial Intelligence (AI) and machine learning (ML) that automate threat detection and response while also ensuring ease of access to networks.
These critical new tools can analyse and identify threats ranging from zero-day vulnerabilities, sophisticated ransomware attacks, and risky behaviour, even from trusted sources, that could lead to a phishing attack or the download of malicious code-based APTs (advanced persistent threats).
While AI and ML are powerful tools for good in the hands of cybersecurity professionals, their malicious use is also the single biggest threat facing digital security today. AI tools can be used by criminals to launch threat vectors with superhuman speed, compromise public utilities and launch automated and targeted disinformation campaigns designed to bring down the morale of society.
Potential misuse
The potential misuse of AI must be factored in when governments and organisations around the world formulate policy, and construct and manage their digital infrastructure. Policymaking at the institutional level is required to ensure the design and distribution of AI systems with safeguards built into these platforms.
AI-based cybersecurity tools stand out because they can be programmed to learn from past attacks to anticipate likely new ones. AI can study behaviour histories, automatically build profiles of users, assets, and networks. This allows them to automatically detect and respond to deviations from established norms.
Traditionally, all AI programmes are “trained” by feeding data to them so that they can recognise patterns to predict future behaviour based on observed “past behaviour” (data). The more data that is fed into the programme the more information it has to predict accurately. That is the reason why AI programmes get better at what they do, as time progresses.
One interesting problem that researchers face while “training” AI programmes for cybersecurity is that, within an organisation, there is usually more data on what can be construed as “normal” behaviour than there is about “abnormal” behaviour.
This presents and interesting set of problems for cybersecurity experts. While past attack patterns could be used as training data, very rarely do hackers repeat the methodology used in a previous attack because they anticipate that a defence has been set up for that type of attack.
AI training
As a result, teaching the AI programmes to distinguish between “good” behaviour and “bad” behaviour doesn’t always work. Instead, what most cybersecurity researchers do is teach the AI to understand what consists of “normal” behaviour for any particular node within the network. Once they are trained on this, the programmes can look out for deviations from this “normal behaviour”.
While most AI programmes have many layers of sophistication built into them, at the most fundamental level, they immediately flag anything different from what should be the normal behaviour. The important point here is that abnormal behaviour may not necessarily mean there is an attack happening on the system.
A simple example of an abnormal but “not malicious” behaviour could be an employee accessing a particular database with a computer which has never been used to access the database. It could very well be the access is due to new work requirements just as it could also mean that the employee’s identity has been compromised and the access is with the intention of data exfiltration.
In an organisation in which several hundred billion signals are bouncing around within the network, the AI programme can instantly flag abnormal behaviour the moment it happens, a feat that is not possible for humans. This allows for quick follow up.
As mentioned earlier, one of the most intractable problems of cybersecurity is that both cybersecurity professionals and hackers have the same access to technology and an equal understanding of how it works. Since AI is usually programmed to flag behaviour that is a deviation from accepted norm, hackers know what the security experts are looking out for and they try to tailor their attack in such a way that it stays within the boundaries of “normal” behaviour to not arouse suspicion.
Cybersecurity professionals on their part understand this and that is why researchers combine Game Theory with AI and ML programmes to second guess the hackers. It boils down to a cat and mouse game.
Scaling up attacks
Just as AI has proved to be an invaluable tool for cybersecurity professionals, it has also made the job of hackers much easier. The ability to scale up operations using AI has allowed many hacking groups to complete tasks that would ordinarily require intelligence and software expertise of the highest order.
Hacking used to be the preserve of highly talented software engineers. With the automation that AI tools allow for, hackers no longer need to even be software engineers.
This has increased the pool of malicious actors as well as the frequency of such attacks because the cost of each attack has gone down. The global spike in cyberattacks witnessed since the outbreak of the novel coronavirus pandemic (Covid-19) last year could at least partly be attributed to the increased automation using AI tools.
Many security researchers believe that the growing use of AI by hackers will allow for more finely targeted and difficult to attribute attacks. There will be no way of knowing which systems are vulnerable and compromised.
To compound matters, this comes at a time when organisations are rapidly becoming digital. Many do not have the wherewithal to keep cybersecurity at the centre of their digital journey.
The government has a major role to play to ensure a safe networked world. Policymakers must collaborate closely with technical researchers to investigate, prevent, and mitigate potential malicious uses of AI.
Like dual-use military technologies, like plane engines and radars, AI policymakers, researchers and scientists need to understand the dual-use nature of their technology. This will ensure that the same standard security protocols, used for dual-use military technologies, also apply to AI-based tools.
This, however, will be easier said than done since much of the cutting-edge research in AI and ML is through open-source collaboration which gives access to all.
A full awareness both from the policy-making side as well as the scientific community is required to ensure that AI remains a force of good and not a tool used to destroy the global digital network which thrives on open access and collaboration. AI researchers and the organisations that employ them are in a unique position to shape the security landscape of the AI-enabled world.
Amit Roy Choudhury, a media consultant, and senior journalist writes about technology for GovInsider.
The region's leading AI innovation forum AI x GOV, powered by GovInsider, is happening on 27 July. Register here.