Artificial intelligence suffers from some very human flaws. Gender bias is one
By Sean Nolan
‘Garbage in, garbage out’ has been a guiding principle of computer technology since its very beginnings, and in the era of artificial intelligence, that hasn’t changed with relation to sexism and other forms of discrimination.
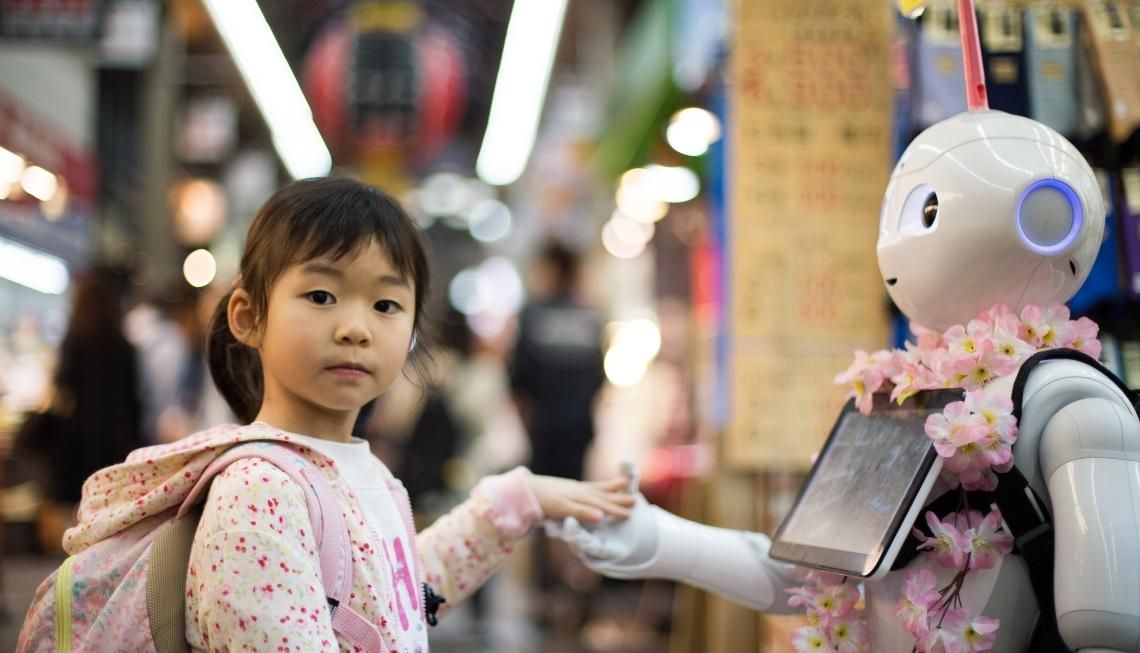
AI, as a mirror of the way in which its creators understand the world, can have biases baked into it. Image: Unsplash/Andy Kelly
BlenderBot 3 is also good at peddling anti-Semitic conspiracy theories, claiming that former US President Donald Trump won the 2020 election, and calling Meta Chairman and Facebook co-founder Mark Zuckerberg “creepy”.
It’s not the first time an AI has gone rogue. In 2016, Microsoft’s Tay AI took less than 24 hours to morph into a rightwing bigot on Twitter, posting racist and misogynistic tweets and praising Adolf Hitler.
Both experiments illustrate the fact that technologies such as AI are every bit as vulnerable to corrosive biases as the humans who build and interact with them. That’s an issue of particular concern to Carlien Scheele, Director of the European Institute for Gender Equality, who says AI may pose new challenges for gender equality.
Scheele says women make up over half of Europe’s population, but only 16 per cent of its AI workers. She says that until AI reflects the diversity of society, it “will cause more problems than it solves”, adding that in AI, limited representation leads to the creation of datasets with inbuilt biases that can perpetuate stereotypes about gender.
A recent experiment in which robots were trained by popular AI algorithms underlines the point. The bots consistently associated terms such as “janitor” and “homemaker” with images of people of colour and women, according to a report by the Washington Post.
Twin challenges
Scheele says two challenges need to be addressed: the immediately pressing task of reducing biases that can be baked into AI, and the longer-term issue of how the diversity of the AI labour force can be increased.
To counter AI bias, the EU has proposed new legislation in the form of the Artificial Intelligence Act, one of whose provisions suggests that AI systems used to help employ, promote or evaluate workers should be considered “high-risk” and be subject to third-party assessments.
The reasoning beyond the provision holds that AI can perpetuate “historical patterns of discrimination” while holding an individual’s career prospects in the balance. Scheele supports the legislation, saying that it can help women pursue their career ambitions through reducing AI discrimination.
She says measures such as the act can tackle biases and discrimination in the short term, but that boosting female representation in AI over the long term is equally important. Scheele says the first step in that direction will be supporting women’s pursuit of science, technology, engineering and mathematics education by countering lazy, counterproductive stereotypes. Without deliberate efforts on the gender integration front, she says, “male-dominated fields will remain male-dominated”.
She also says that businesses and other entities using AI should encourage increased representation of women in order to ensure “a fuller spectrum of perspective”, because a more inclusive perspective will foster the development of skills, ideas and innovations that measurably benefit their performance.
Abby Seneor, Chief Technology Officer at Spanish social data platform Citibeats, says that increasing the diversity of those working in AI is crucial, because when AI systems are being developed, a human “decide[s] whether the output of this algorithm is right or wrong, and that’s purely down to the engineer”. The involvement of people with not only the right qualifications, but who can also identify biases, is therefore critical, she says.
Open source community
Another means of tackling AI bias is sharing AI models with others, Seneor says, pointing to the “ethical AI community” of like-minded organisations that Citibeats works with.
Citibeats provides input to governments by gauging public sentiment on various issues through monitoring social media content using natural-language processing and machine learning. It shares information with other organisations that maintain their own datasets so it and its collaborators can test AI models and report potential biases or faults to developers.
If for example, a team is developing an AI model to scan photos and identify people’s genders, they could find themselves limited by the fact that they may be working only in one part of the world. But by sharing their model with organisations elsewhere, they can test their model using images of a greater range of human subjects, contributing to the effectiveness of the AI model.
Seneor says creating unbiased AI is not a job only for practitioners, but also for policymakers, who she says “need to get up to speed with the technology” and would benefit from more engagement with people involved in AI at a practical level.
Stanford University seeks to foster this kind of engagement, and last month invited staff from the US Senate and House of Representatives to attend an “AI boot camp” at which AI experts explained to them how the technology would affect security, healthcare and the future of work.
Seneor also supports more regulation of big tech companies involved in AI, such as DeepMind, owned by Google parent Alphabet, because the algorithms they create affect millions of people. “With this big power comes big responsibility,” she says.
Regulation could mandate that big tech companies be open about how their AI works and how it may change. It may also demand that AI models be tested with greater transparency, which would represent a significant departure from the secretive way in which businesses in the field currently operate. Seneor says companies embed AI in products that everyone uses, but that people “have no idea what's going on inside”.
AI in the gig economy
The European Institute for Gender Equality says the gig economy is one sphere in which AI can lead to unfair outcomes for women. AI algorithms often determine workers’ schedules on platforms such as Uber and Deliveroo, according to a report it published at the beginning of the year. The algorithms use data such as employment history, shift changes, absences and sick leave to allocate new tasks and evaluate performance, potentially leading to unequal treatment of women, whose work histories can be complicated due to maternity and other commitments. In a study of 5,000 gig workers, the institute found one in three took on gig work while balancing family responsibilities and housework.
Scheele says that although addressing unfair AI is key, governments can play a part in creating “a gig economy that works for women” by ensuring that workers have access to a strong social security system. She says providing health and life insurance, pension schemes and maternal support can give women “the psychological safety of knowing there is a net to catch them” if something unexpected happens in the course of gig work.
As the world continues its digital transformation, breakthrough developments in technology beckon, offering much potential to improve people’s lives. But it’s important to recognise that technology is never completely agnostic, and that biases and discrimination can be baked into it as much as they can any other human creation. That makes it all the more important that technological development, of which AI is an increasingly important part, be informed by considerations of non-discrimination and fairness if all the growing momentum of digital innovation is to bear fruit equitably.