How AI helps finance improve inclusion and predict future trends
By Liew Ming En and Sean Nolan
Iota Kaousar Nassr, Economist / Policy Analyst, Directorate for Financial and Enterprise Affairs, OECD delves into the perks and risks of using AI and ML in the financial sector.
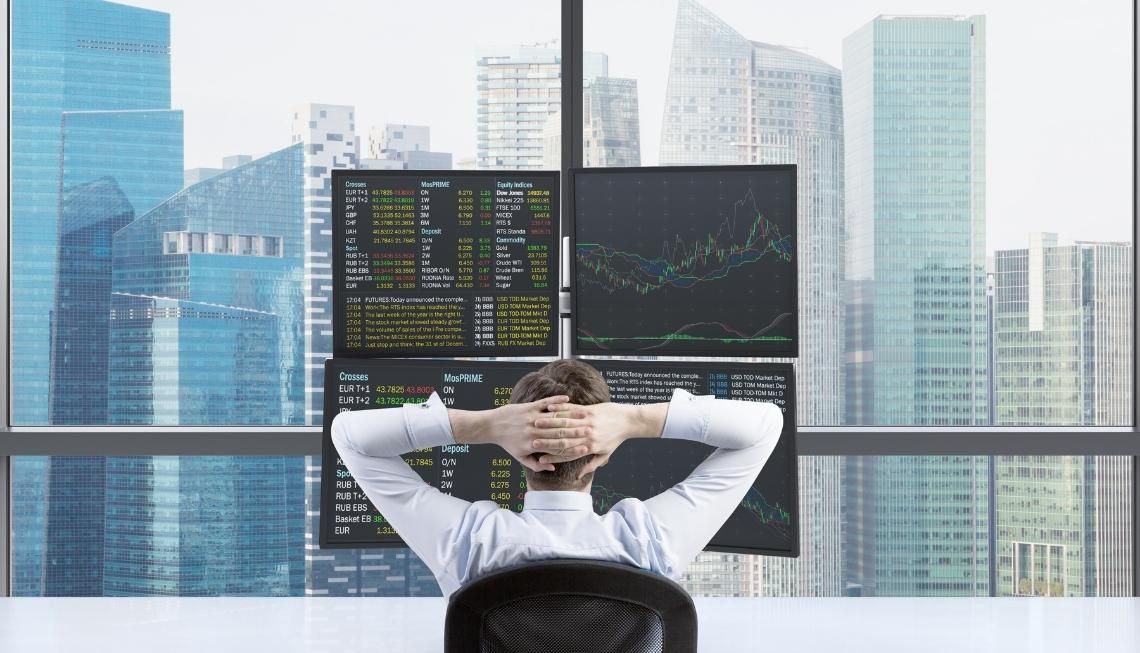
But the future as predicted by a crystal ball can be murky. As much as the perks of using AI in the financial sector are plentiful, there are substantial risks associated with it.
Iota-Kaousar Nassr, Economist/Policy Analyst, Directorate for Financial and Enterprise Affairs at the Organisation for Economic Co-operation and Development (OECD), explores the upsides of using AI and machine learning (ML) in the financial sector. She also provides insights on how policy makers can best avoid their pitfalls.
Financial inclusion for small businesses and citizens with AI
AI can improve financial inclusion by helping banks determine the credit score of citizens and micro businesses, she elaborates. Credit scores affect banks’ decisions to lend money to citizens and businesses.
Citizens or young SMEs with a limited credit history often have difficulty accessing financing, Nassr says. AI can draw on social media data or internet history to determine their ability to repay a loan.
By alleviating the constraints to SME financing, financial institutions can better support their access to finance and the growth of the economy. This “may be one of the most transformational use cases in finance,” says Nassr.
How ML predicts financial markets more accurately
ML models in finance draw from big data to make more accurate predictions about the market. They monitor thousands of risk factors daily and simulate possible investment performance against thousands of market and economic scenarios. This lowers the investment risks for financial institutions and citizens.
AI also helps investors draw insights from more places to inform their investment strategies within a short timeframe. The algorithms can process unstructured data such as images and voices as opposed to just numbers.
A growing body of research finds that AI-based investments are outperforming traditional ones. Likewise, a study found that analysing how newspapers talk about the economy with ML can reliably predict how an economy is doing through factors like inflation and GDP.
The risks of AI and ML
AI and ML have the potential to improve efficiency and inclusion, but they bring two main risks.
First, AI-based credit scoring models may cause discriminatory or unfair lending, highlights Nassr. While a credit officer may be careful not to include gender- or race-based factors in their scoring, ML might unknowingly take such factors into account.
That’s because ML models are only as reliable as the data they are trained with. Models that are trained with poorly labelled data, or data that reflects underlying human prejudices, may produce inaccurate results even when later fed with ‘good’ data, says Nassr.
Second, algorithms can also make financial institutions more vulnerable to cyber attacks. It’s easier for cyber criminals to exploit models that are all acting the same way, as opposed to human-led systems which act independently.
Navigating the pitfalls of AI and ML
Policymakers need to ramp up their existing arsenal of defences against the risks associated with AI and new technology, says Nassr. One way they can do so is through clear communication.
For instance, financial institutions should inform users if a service uses AI. They should also clearly state the limitations of AI models so that users can make informed financial decisions, explains Nassr. This helps instill trust and confidence, and promotes the safe adoption of tech like AI.
Additionally, policy makers should emphasise human decision making over AI-led ones. This is especially so for higher-value use cases such as money lending which can have a significant impact on citizens’ lives.
Citizens can be empowered in this situation with processes that allow them to challenge the outcome of AI models, and seek compensation where necessary. Citizens could also be given the option to opt-out of having their data analysed in AI models.
Over time, these measures promote trust in new tech like AI and ML.
It is also important for policy makers to place a greater emphasis on prudent data management and usage, highlights Nassr.
Policy makers should ensure that financial institutions test AI and ML models before they are used, in order to avoid potential biases. This also ensures that the models are operating as intended and adhere to any existing rules and regulations.
AI and ML can help financial institutions develop a more accurate forecast of financial markets, but it is no magic crystal ball. Rather, they are tools with immense potential, if their pitfalls are safely addressed and navigated.