This scientist used analytics to fight child lead poisoning
By Nurfilzah Rohaidi
Exclusive interview with Rayid Ghani, Director of the Centre for Data Science and Public Policy at the University of Chicago.
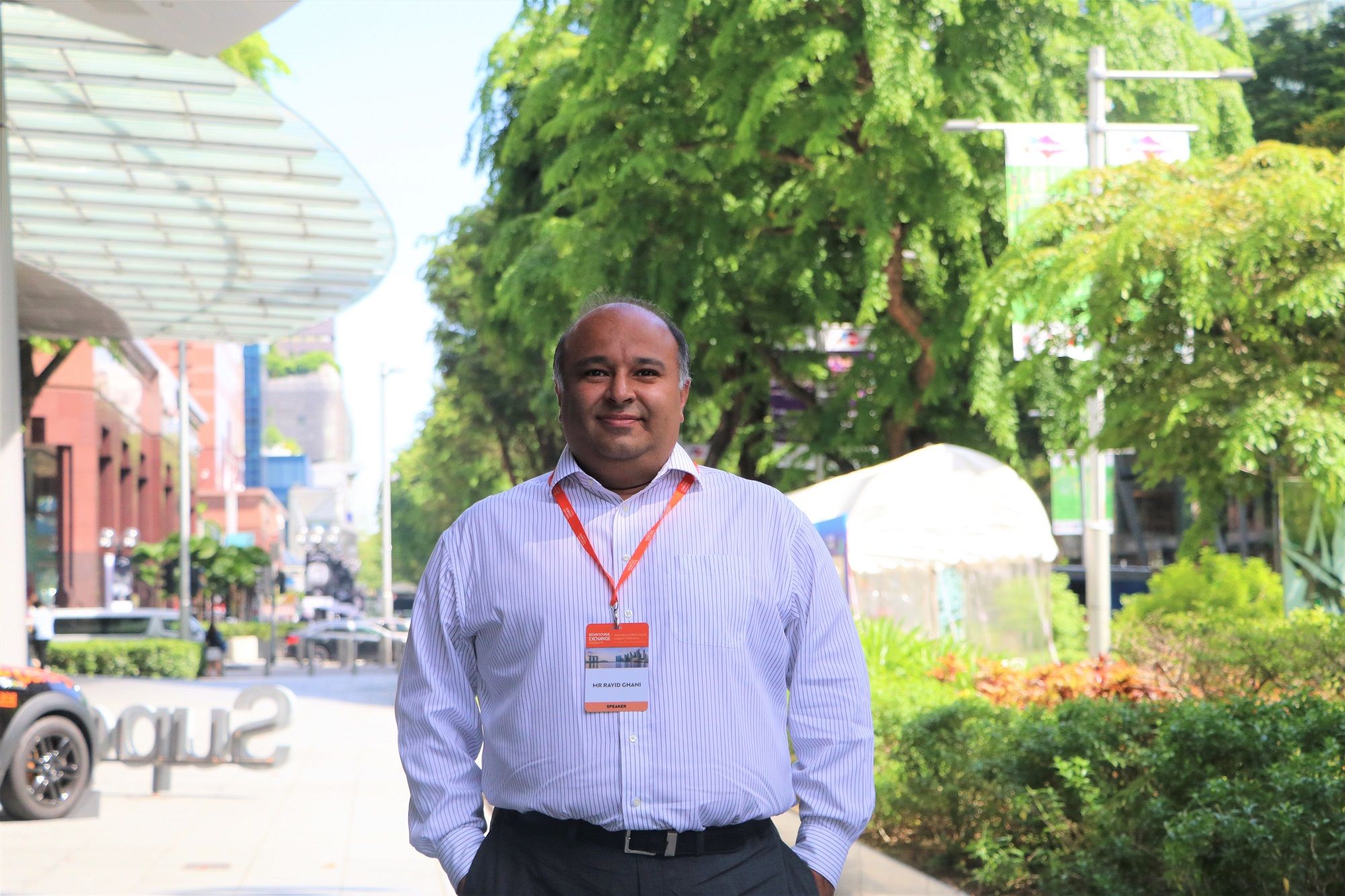
Every American home built before 1978 has lead in its walls, which can flake off and be eaten by young children. Accurate housing data can therefore be used by doctors to predict whether children are at risk of lead poisoning, says Rayid Ghani, Director of the Centre for Data Science and Public Policy at the University of Chicago.
Speaking on the sidelines of the Behavioural Exchange 2017 conference, he explains how predictive services have prevented irreversible harm to vulnerable young people, reduced suicide risks, and helped in law enforcement.
Poison in the walls
Ghani’s team first began trials of the predictive system in Chicago last August, and over the next few weeks, the system will be introduced across the city. He adds, “We’ll also be implementing a hospital system so that when a pregnant woman goes in for a checkup, it can predict if the unborn child will be at risk.”
According to the Centre for Disease Control, children in at least four million households across the US are being exposed to high levels of lead. There are approximately half a million US children aged 1-5 with blood lead levels higher than normal.
What are some of the indicators that a child may be at risk of lead poisoning? Parents’ behaviour plays a role: “Do they wash their kid’s hands all the time? Do they sweep the floor? Do they have paint chipping from their walls?”
But understandably, some of this data may not be available, or difficult to collect—you obviously cannot have cameras in these homes, says Ghani—so “you look for proxies to help you identify that behaviour”, he explains. “We take anything we know about the kid, parents and house,” Ghani says. This includes when the house was built, its location, how many apartments and floors it has, and whether there were any incidents nearby. This data allows the system to predict a child’s risk of poisoning in a particular home.
This is an intervention, officer
Another social problem that Ghani is working on is the unjustified use of force by the police—a hot-button topic at the moment.
The Guardian reported that in 2015, young African-American men were nine times more likely than other Americans to be killed by police officers, and there were a total of 1,134 deaths at the hands of law enforcement that same year. “Police departments have had a lot of incidents with citizens where they’ve had to use unjustified force, shootings that shouldn’t have happened,” Ghani says.
Similar to the system used to predict risk of lead poisoning, Ghani partnered with police departments to build a system to predict the future risk of an officer acting in this manner. “We provide information to the police department so that they can intervene with counselling to reduce the chances of that happening,” he says.
“We provide information to the police department so that they can intervene with counselling to reduce the chances of that happening.”Ghani’s analysis shows that officers are more likely to be aggressive if they have been assigned to particularly stressful cases. Two “really big predictors” are if the officers were recently assigned to domestic abuse cases, especially involving children, and suicide attempts, he explains.
Such insights allow police departments who have limited resources to prioritise certain officers. Ghani’s system has reduced the number of false positives by 50%, and identified 20% more at-risk officers using machine learning and prediction algorithms. It will be deployed in Charlotte, North Carolina this summer, and will be expanded to Pittsburg, San Francisco to “see if that actually works across the cities”.
Ins and outs of the system
A third system that is in the prototype stage identifies people who are at risk of being repeat offenders, a phenomenon called recidivism. A 2016 study by the US Sentencing Commission revealed that in 2005, nearly a third of 25,400 inmates released from prison or placed on probation were reconvicted within that same year, with 24.7% of them also re-incarcerated.
According to Ghani, two-thirds of the individuals in the jail and prison systems have “health problems, mental health issues, chronic health issues, substance abuse problems”. Often, these people are stuck in a vicious cycle, going in and out of mental health facilities, homeless shelters, social services, or back to jail.
Ghani is combining information from these facilities to identify repeat offenders early on, so that the relevant departments may act. “We can intervene and tell the homeless shelter, ‘This person is coming to you, they need a shelter but they’re also at risk of going to jail in the next year. Can you please make sure you spend time with them, whatever it takes?’”
He adds that in the system’s top 200 predictions, 104 of those individuals went to jail in the next year. Now, he is working with Johnson County, Kansas to provide mental health co-responders and case managers with a list of individuals in need of support.
Data can help to alleviate traffic woes, speed up commutes and slash operating costs. But sometimes, it can inspire real change in people’s lives. How could it be used in your government?