From data to delivery: How GenAI is shaping the future of public service
By SAS
GenAI initiatives should support broader public goals and needs, says global AI and analytics firm SAS’ Ensley Tan.
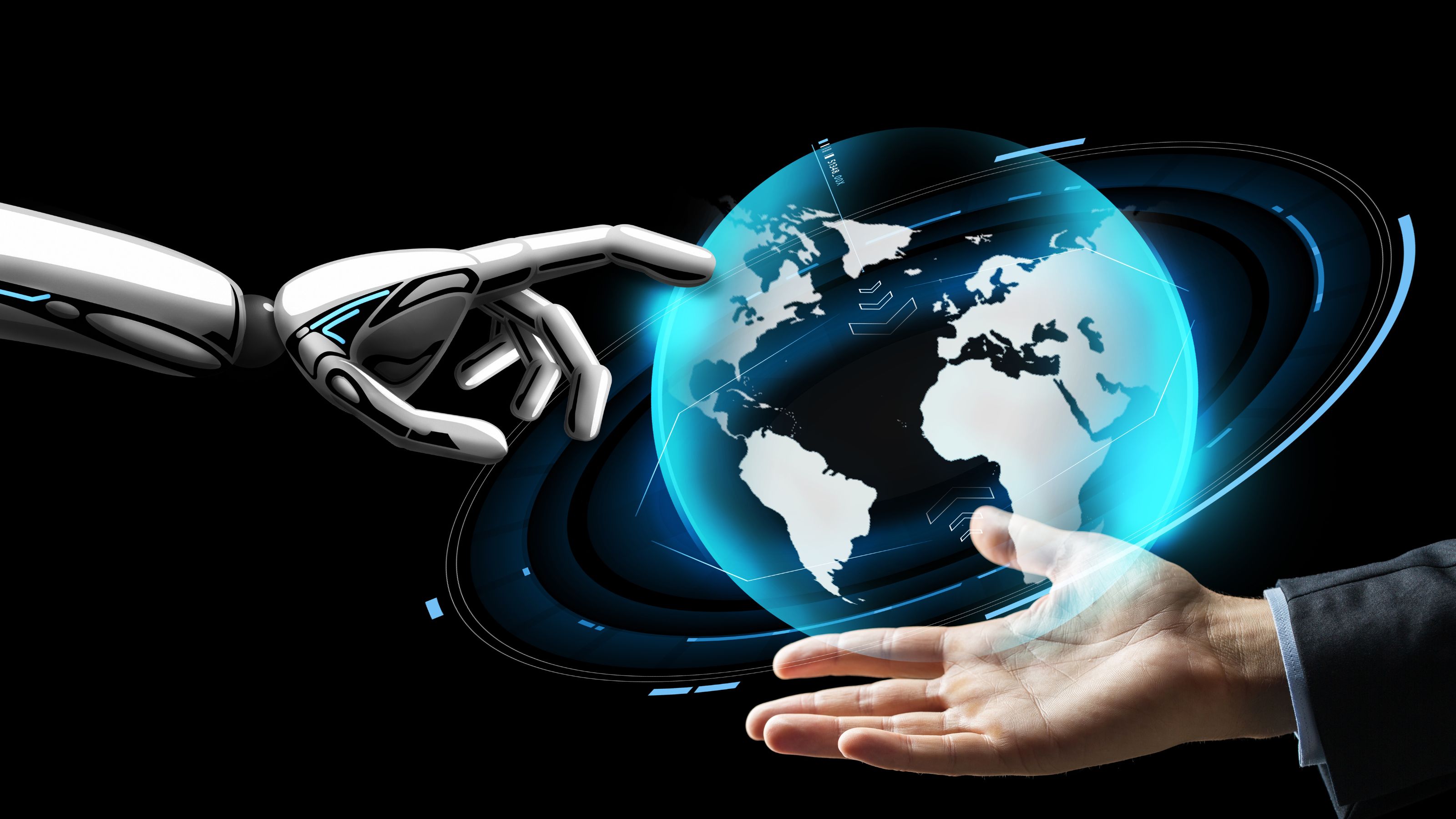
Public sector agencies should gauge the success of their GenAI initiatives by focusing on their existing key performance indicators, says SAS' Ensley Tan. Image: Canva.
While governments recognise the potential of generative AI (GenAI) to improve operational efficiency and citizen experience, there is more to it than just setting up projects and simply expecting them to work.
SAS is a global leader in artificial intelligence (AI) data analytics that supports diverse industries such as finance, pharmaceuticals and governments.
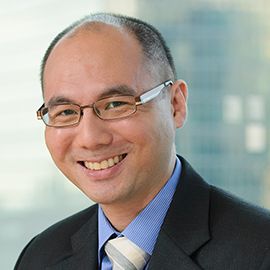
Its Asia-Pacific Lead for Public Sector Consulting, Ensley Tan, said public sector agencies should gauge the success of their GenAI initiatives by focusing on their existing key performance indicators that are supported by GenAI.
In other words, they should be tracking how GenAI solutions are helping to optimise service delivery and support the agency’s missions.
“GenAI efforts should always be linked to real-world goals and needs (such as better quality of life, financial growth, national security or simple internal efficiencies), and not pursued in and of themselves,” he explained.
Tan shared a few potential use cases for GenAI in the government, top challenges faced in scaling up the technology, as well as potential ways to overcome those challenges.
To subscribe to the GovInsider bulletin click here.
GenAI’s potential beyond chatbots
Beyond chatbots and customer service, Tan highlighted two underutilised areas where GenAI could revolutionise public services.
One of them is in policy and legislation research. Currently, such research tends to be a manual process, said Tan, and policymakers find it difficult to keep track of all the global developments and impacts that might be relevant to a particular policy.
Large-language models (LLMs), a type of GenAI that can be good at interpreting complex search queries, is one way to quickly comb through policy documents for relevant areas of reference for policymakers.
Notably, combining LLMs with either predictive analytics or digital twins will allow policymakers to simulate the outcomes of different policy options, Tan said. An example of this is Singapore’s JTC Corporation (JTC), which oversees smart city developments and is experimenting with LLM-integration with digital twins.
Generative adversarial networks (GANs) are another type of model that enables governments to tap on GenAI innovation in a secure and controlled manner, he added.
GANs contain two key components – a generator and a discriminator. The generator creates synthetic data like images or text, while the discriminator assesses its authenticity by comparing it to real data.
Essentially, the generator produces data that tries to look real, and the discriminator measures how real it looks. As both components improve, the generator produces increasingly realistic data.
Within the public sector, Tan said that GANs are being used to generate artificial data. Such data is currently being used in areas with data limitations, like healthcare, to enable clinical research and medical education without compromising patient privacy.
To subscribe to the GovInsider bulletin click here.
Tackling cost, LLM’s liability and security
Tan said the three top obstacles faced in scaling GenAI adoption in the government are security, cost, and the reliability of LLMs.
1. Security concerns
Internally hosted LLMs tend to be the primary approach for the public sector, as agencies are reluctant to share their data, queries, or results with third parties, Tan added.
Internally hosted LLMs will enable organisations to retain full control over all aspects of LLM usage.
However, if an organization only uses its internal data for training, the data may be limited in quantity and diversity, which can restrict the LLM’s analytical capabilities.
2. High cost
Another challenge is the high cost of hosting, training and maintaining LLMs, and organisations will need to justify the cost with meaningful use cases.
Tan thought that one way to address this constraint is to consider a whole-of-government LLM, similar to the move towards government-hosted clouds.
3. Reliability concerns
As for the unreliability in LLM responses, a mix of prompt engineering, and evaluation over time might solve this issue but it remains an evolving space, he said.
Prompt engineering involves crafting very specific inputs to improve the reliability and transparency of LLM responses, while long-term evaluation focuses on ongoing monitoring and adjustments to enhance performance.
On this note, JTC is also currently training AI systems with officially sanctioned datasets to lower the risks of AI hallucinations (i.e., generating inaccurate or false information) for its smart city project.
Human in the loop, explainability will be key
A key issue here is that it is important to have a human in the loop when using GenAI for decision-making. So GenAI outputs should be explainable.
“A human in the loop will be the first defence for the public sector in trying to develop the public’s trust in AI,” he emphasised.
However, explainability is not unique to GenAI. As with other forms of AI, explainability is also crucial to scale up the adoption of the technology, he explained.
“For GenAI, explainability can range from simply listing the sources used in the response, to relying on more trusted AI models to generate the conclusions upon which the LLM’s response is based,” he noted.
Compared to traditional AI tools which tend to require more sophisticated skills to use, GenAI will probably have a “lower skill bar to use,” said Tan. But layman users will still have to understand its potential and pitfalls.
Public-private partnerships can help provide a hybrid approach to skills development. Digital government research and development (R&D) centres can collaborate with GenAI leaders from the private sector to keep up with the rapidly evolving state of GenAI and its related skillsets.
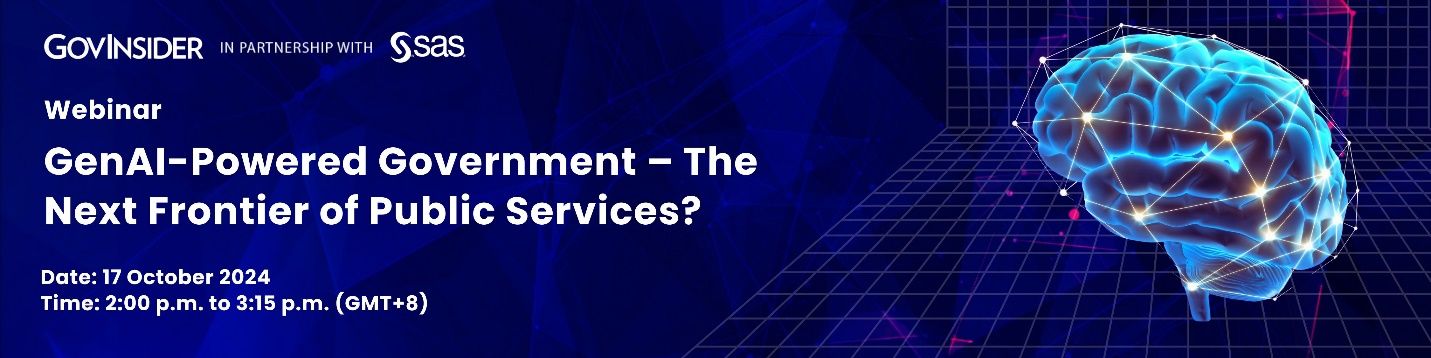