Why machine learning is the future of human resources
By Workday
Sandeep Sharma, President of Workday Asia, shares how data analysis can save time and resources.
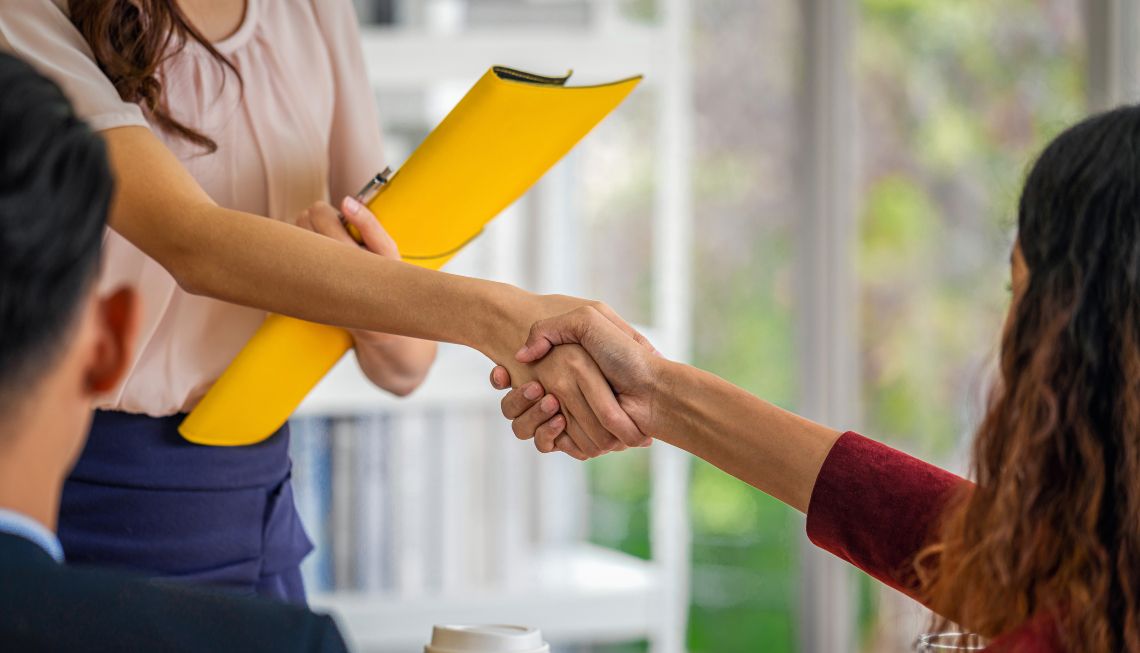
Organisations must ensure that talent among their workforce is not being overlooked.The human resources team is always looking to promote and employ the best and brightest. Machine learning makes identifying ideal candidates easier.
There is currently a “war for talent”, says Sandeep Sharma, President of Workday Asia. It is vital that human resources management can keep up with other areas of an organisation. He shares why machine learning is the tool to do that.
Identifying talent within the workforce
The public sector “is one of the largest employers in any economy”, states Sharma. Managers in any large organisation face the common challenge to keep track of the progress every employee is making.
Machine learning can help. For instance, global biopharmaceutical company AstraZeneca used Workday’s tools to recognise skill gaps in its over 65,000-strong workforce.
The tools provided “useful insights” for managers by analysing trends and recognising patterns, Sharma explains. This allowed internal promotions or hiring to be done according to the company’s unique needs.
Machine learning has saved AstraZeneca time, resources and money, shared Liz Moran, the firm’s Global Head of Talent. It “enables us to do things in the past we could have only dreamed of”, she added.
Machine learning can help managers to “identify career tracks” as well, says Sharma. Data analysis provides “evidence-based predictions for future performance”, which is free from managers’ potential bias, he adds.
Clothing brand Patagonia reported that after adopting machine learning, employee performance data went up by 45 per cent. They were then able to “figure out creative ways to create career advancement”, said Shannon Ellis, their Director of Human Resources.
Making hiring more efficient
Sharma identifies two ways machine learning can help with assessing potential candidates. Firstly, the system can “map out a large number of job applications”, making it easier for recruitment teams to sift through.
Secondly, analytics can sort out resumes based on key skills and qualifications. For the public sector, which has “extensive requirements on background and skillsets”, machine learning can speed up this process, states Sharma.
In terms of long-term planning, organisations looking to grow should have an idea of how many people will be required and in what positions. Workday can help companies prepare for uncertain futures through employment planning models, Sharma said.
These planning systems allow for scheduled hiring of new people, recognising of unfilled positions and can take note of employees contracts’ whether they be full time or part time.
Workday helped a global financial services and communications company create a plan to manage its workforce over the next three years, says Sharma. Workday took on many of these tasks as organisations struggled to deal with manpower issues caused by Covid-19, he adds.
Managing large quantities of data
Like finding a needle in a haystack, many governments are tasked with searching for value amongst a sea of available data.
Over 2.5 quintillion bytes of data are created every single day, according to a report by Domo. It was estimated that in 2020,1.7MB (enough to store 30 seconds of your favourite song) was created every second for every person on earth.
Dealing with such massive amounts of data is where machine learning “truly shines”, says Sharma. As their name implies, these machines learn how to process data through training, eventually making them faster than human employees or other basic computing algorithms.
“Cumbersome legacy systems” is a challenge Sharma identifies in the public sector. Incorporating machine learning analysis should be part of a wider move into new data-driven systems, he recommends.
Ensuring data is reliable
Machine learning “excels at identifying what matters and making accurate predictions”, but there is still a need for human interpretation, admits Sharma. In his experience, technology brings both advantages and issues when it interacts with human data managers.
On one hand, data analytics can help to identify and reduce biases that humans bring. But programming issues could mean bias is spread across all the machine’s tasks.
Data programmers must decide what “fairness” should mean for the system, suggests Sharma. Preparing data properly and frequent reviews of the benchmarks for “fairness”, help to address potential biases in AI, he explains.
Machine learning can sift through employee and recruitment data, faster and more effectively than a human could. In a competitive world of finding the best talent, organisations should seize every advantage to prevent lagging behind their rivals.